Artificial intelligence (AI) is transforming the way we conduct scientific research. From automating experiments to predicting outcomes, AI is enabling researchers to perform tasks that were previously impossible or impractical. In this blogpost, we will explore five ways researchers are harnessing machine intelligence in the laboratory and how it is accelerating discoveries in various fields of study. Without further ado, let’s delve into the exciting ways AI is revolutionizing scientific research!
Automating Experimentation
One of the ways researchers are using AI is to automate repetitive and tedious laboratory tasks. For example, AI can be used to control robotic systems that can prepare and analyze samples, such as blood, tissue, or chemical compounds. This can save time and resources, as well as improve the reproducibility and accuracy of the experiments.
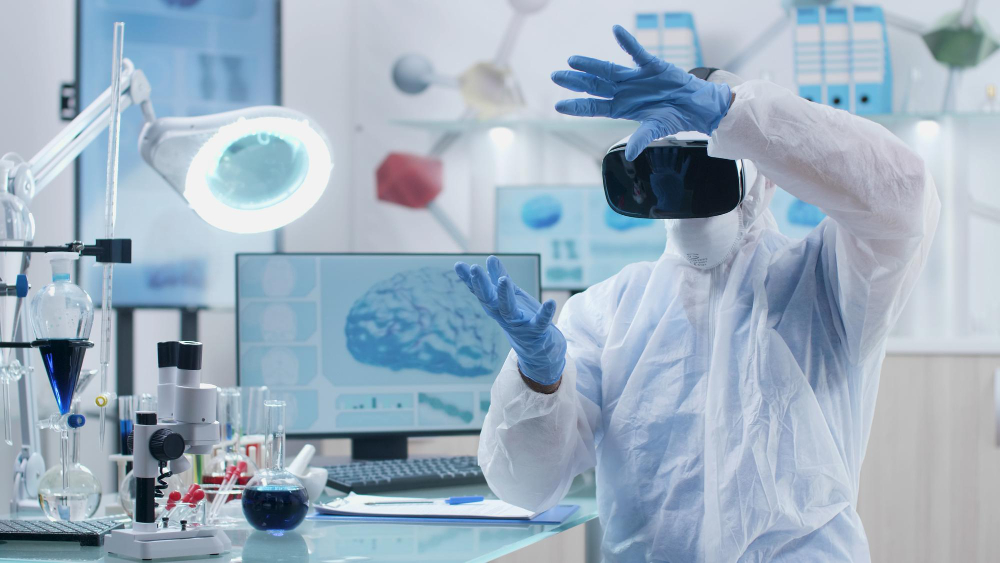
Some examples of AI-driven robotic systems for laboratory automation are:
- RoboRXN, a cloud-based platform developed by IBM that can synthesize organic molecules on demand using a robotic arm and a deep learning algorithm.
- Eve, a robot scientist designed by researchers from the University of Manchester and Aberystwyth University that can screen potential drugs for malaria and other diseases using a combination of machine learning and automated reasoning.
- Ada, a robotic system developed by researchers from the University of California, Berkeley and the University of California, San Francisco that can perform high-throughput microscopy and image analysis for studying cellular dynamics.
By using AI to automate experimentation, researchers can increase the efficiency and quality of their work, as well as reduce human error and bias.
Predictive Modeling
Another way researchers are using AI is to create predictive models that can simulate and forecast the behavior of complex systems. For example, AI can be used to predict the outcomes of chemical reactions, the structures of proteins, or the properties of materials. This can help researchers generate new hypotheses and guide their experimental design.
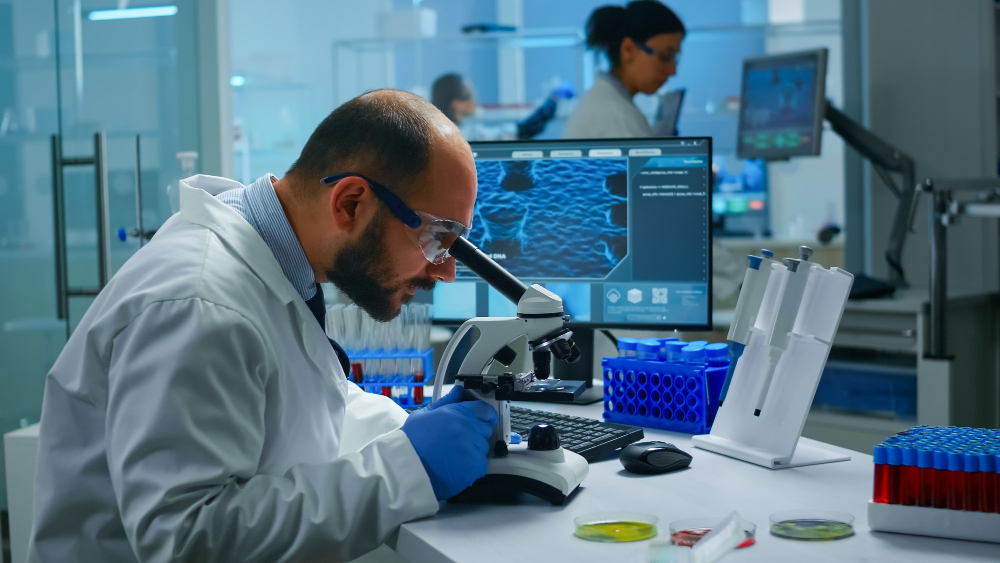
Some examples of AI algorithms for predictive modeling are:
- IBM RXN, a web-based tool that can predict the products and yields of organic reactions using a neural network trained on millions of chemical reactions.
- AlphaFold, a deep learning system developed by DeepMind that can predict the three-dimensional structure of proteins from their amino acid sequences with unprecedented accuracy and speed.
- SchNet, a neural network developed by researchers from the Technical University of Berlin and the Fritz Haber Institute that can predict the properties of molecules and materials from their atomic configurations.
By using AI to create predictive models, researchers can explore new possibilities and discover new insights that would otherwise be difficult or impossible to obtain.
Data Analysis and Pattern Recognition
A third way researchers are using AI is to analyze and interpret large and complex datasets. For example, AI can be used to identify patterns and correlations in genomic, proteomic, or metabolomic data, or to classify and segment images from microscopy, radiology, or astronomy. This can help researchers extract meaningful information and knowledge from their data.
Some examples of AI techniques for data analysis and pattern recognition are:
- DeepVariant, a deep learning tool developed by Google that can accurately identify genetic variants from sequencing data.
- CellProfiler, an open-source software developed by researchers from the Broad Institute and Harvard Medical School that can quantify and measure features of cells from microscopy images using machine learning.
- AstroNet, a convolutional neural network developed by researchers from Google and Harvard University that can detect and classify exoplanets from light curves using data from the Kepler space telescope.
By using AI to analyze and interpret data, researchers can enhance their understanding and explanation of complex experimental results.
Drug Discovery and Development
A fourth way researchers are using AI is to accelerate drug discovery and development pipelines. For example, AI can be used to screen and rank potential drug candidates, to optimize their chemical structures and properties, or to evaluate their safety and efficacy. This can help researchers reduce the time and cost associated with drug development, as well as increase the success rate and quality of the drugs.
Some examples of AI-driven approaches for drug discovery and development are:
- DeepMind, a research company that used its AlphaFold system to predict the structures of several proteins associated with COVID-19, which could facilitate the development of new treatments and vaccines.
- BenevolentAI, a biotechnology company that used its AI platform to identify an existing drug, baricitinib, as a potential treatment for COVID-19, which was later tested in clinical trials and approved for emergency use.
- Atomwise, a startup company that used its AI technology to discover new drugs for various diseases, such as Ebola, multiple sclerosis, and Alzheimer’s, by screening billions of molecules for their binding affinity and selectivity.
By using AI to accelerate drug discovery and development, researchers can improve the efficiency and effectiveness of their work, as well as address unmet medical needs and challenges.
Personalized Medicine and Healthcare
A fifth way researchers are using AI is to apply personalized medicine and healthcare. For example, AI can be used to diagnose and treat patients based on their individual characteristics, such as their genetic profile, medical history, or lifestyle. This can help researchers optimize the outcomes and quality of life of the patients, as well as prevent or delay the onset of diseases.
Some examples of AI algorithms for personalized medicine and healthcare are:
- Watson for Oncology, an AI system developed by IBM that can provide personalized and evidence-based recommendations for cancer treatment based on the patient’s genomic data and clinical records.
- DeepMind Health, a research initiative by DeepMind that aims to use AI to improve the diagnosis and management of various health conditions, such as eye diseases, kidney injuries, or head and neck cancers.
- Ginger, an app that uses AI to provide personalized and on-demand mental health care, such as coaching, therapy, or psychiatry, based on the user’s mood, behavior, and needs.
By using AI to apply personalized medicine and healthcare, researchers can revolutionize the delivery and accessibility of health services, as well as improve the well-being and satisfaction of the patients.
Conclusion
In this blogpost, we have explored five ways researchers are harnessing AI in the laboratory and how it is accelerating discoveries in various fields of study. AI is not only a powerful tool, but also a collaborative partner for researchers, as it can automate, predict, analyze, discover, and personalize their work. As AI continues to evolve and improve, we can expect to see more breakthroughs and innovations in scientific research, as well as more benefits and impacts for society and humanity.