Introduction
The universe is a vast and mysterious place, full of wonders and enigmas that have fascinated humans for centuries. From the origin of the cosmos to the nature of dark matter and dark energy, there are many questions that remain unanswered by conventional science. Can artificial intelligence (AI) help us unravel these cosmic mysteries? How far can AI go in advancing our understanding of the universe?
AI is a branch of computer science that aims to create machines and systems that can perform tasks that normally require human intelligence, such as learning, reasoning, and problem-solving. AI has revolutionized various fields, such as medicine, education, and entertainment, by enhancing human capabilities and creating new possibilities. AI has also made significant strides in astrophysics and cosmology, the scientific disciplines that study the structure and evolution of the universe.
In this blog post, we will delve into the capabilities and limitations of AI in astrophysics and cosmology. We will explore how AI is being utilized in various aspects of astrophysical research, such as data analysis, image recognition, and simulations. We will also highlight the advantages of AI, such as its ability to process large datasets, identify patterns, and accelerate scientific discoveries. We will provide examples of breakthroughs made possible by AI in astrophysics, showcasing instances where AI has outperformed traditional methods. We will also discuss the challenges and limitations of AI in dealing with the sheer volume and complexity of astronomical data, as well as the ethical implications of relying too heavily on AI in scientific research. Finally, we will consider the future of AI in astrophysics, advocating for a collaborative approach that combines the strengths of AI with human expertise, and exploring the potential for hybrid models that integrate AI algorithms with human intuition and creativity. We will also speculate on the possibilities of AI in pushing the boundaries of our understanding of the universe, while remaining grounded in realistic expectations.
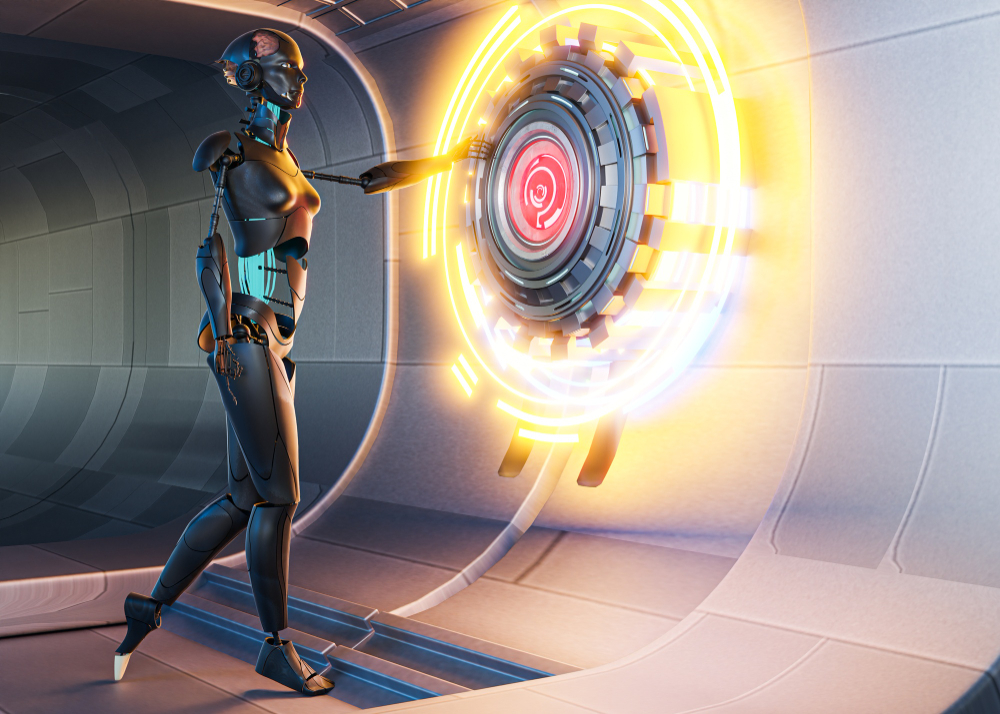
Understanding the Cosmos
The universe is estimated to be around 13.8 billion years old, and contains more than 100 billion galaxies, each with billions of stars and planets. The observable universe, the part of the universe that we can see with our telescopes, spans about 93 billion light-years in diameter. However, the observable universe is only a tiny fraction of the whole universe, which may be infinite in size and contain multiple dimensions and parallel universes.
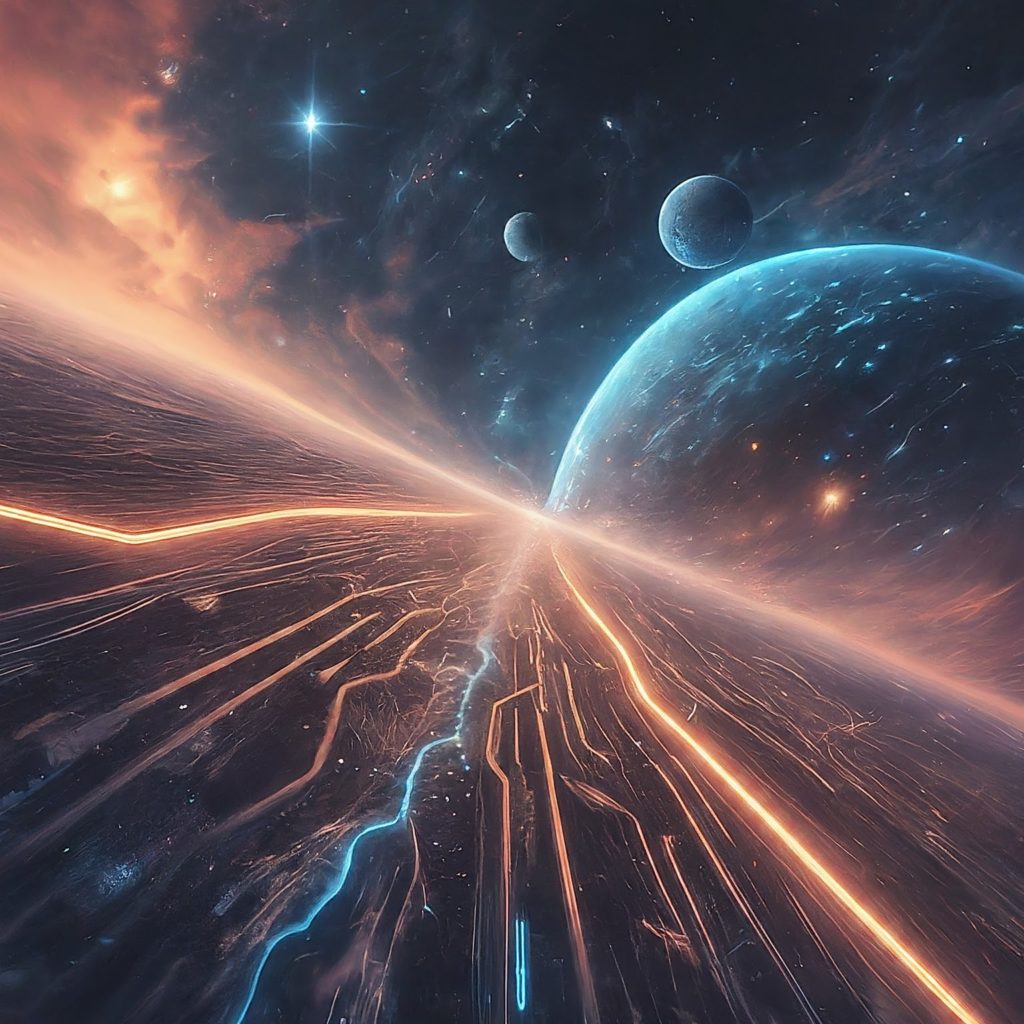
The universe is also full of mysteries that challenge our current understanding of physics and reality. For instance, we do not know what most of the universe is made of. About 95% of the universe is composed of dark matter and dark energy, two mysterious substances that we cannot see or detect directly, but only infer from their gravitational effects on the visible matter. We also do not know how the universe began, or how it will end. The most widely accepted theory is the Big Bang theory, which states that the universe started from a singularity, a point of infinite density and temperature, and then expanded and cooled down over time.
However, the Big Bang theory does not explain what caused the singularity, or what happened before it. Moreover, the fate of the universe depends on the balance between its expansion and contraction, which is determined by the amount and nature of dark energy. The universe may continue to expand forever, or eventually collapse into a Big Crunch, or rip apart in a Big Rip.
Humans have always been curious about the universe, and have tried to understand it through observation and experimentation. Astronomers and cosmologists have used various instruments, such as telescopes, satellites, and probes, to collect and analyze data from the universe, such as light, radio waves, and gravitational waves. They have also developed mathematical models and theories, such as general relativity and quantum mechanics, to explain and predict the behavior of the universe. However, these methods have their limitations, as they are constrained by the availability and quality of data, the accuracy and completeness of models and theories, and the computational and cognitive resources of humans.
The Rise of AI in Astrophysics
AI has emerged as a powerful tool in astrophysics and cosmology, as it can augment and complement the traditional methods of scientific research. AI can be applied to various aspects of astrophysical research, such as data analysis, image recognition, and simulations.
Data Analysis
One of the main challenges in astrophysics and cosmology is the analysis of large and complex datasets, which are often noisy, incomplete, and heterogeneous. AI can help in this task by using machine learning, a subfield of AI that enables machines to learn from data and improve their performance without explicit programming. Machine learning can be used to perform tasks such as data cleaning, feature extraction, dimensionality reduction, clustering, classification, regression, and anomaly detection.
For example, machine learning can be used to clean and calibrate the data from the Large Synoptic Survey Telescope (LSST), a project that aims to survey the entire southern sky every few nights for a decade, producing about 20 terabytes of data per night. Machine learning can also be used to extract features from the data, such as the shape, size, color, and brightness of astronomical objects, and reduce the dimensionality of the data, such as by using principal component analysis (PCA) or autoencoders. Machine learning can also be used to cluster the data into groups of similar objects, such as galaxies, stars, and asteroids, and classify the data into categories, such as supernovae, quasars, and gravitational lenses. Machine learning can also be used to perform regression, such as by estimating the distance, mass, or age of astronomical objects, and anomaly detection, such as by identifying rare or unusual events, such as gamma-ray bursts, fast radio bursts, or alien signals.
Image Recognition
Another challenge in astrophysics and cosmology is the recognition and interpretation of images, which are often blurry, distorted, or obscured by noise or interference. AI can help in this task by using computer vision, a subfield of AI that enables machines to understand and manipulate images and videos. Computer vision can be used to perform tasks such as image enhancement, segmentation, detection, recognition, and generation.
For example, computer vision can be used to enhance the images from the Hubble Space Telescope, which are often affected by atmospheric turbulence, optical aberrations, and cosmic rays. Computer vision can also be used to segment the images into regions of interest, such as galaxies, stars, and planets, and detect the boundaries and locations of these regions. Computer vision can also be used to recognize the objects in the images, such as by using convolutional neural networks (CNNs), a type of machine learning model that can learn to identify complex patterns in images. Computer vision can also be used to generate new images, such as by using generative adversarial networks (GANs), a type of machine learning model that can learn to create realistic images from noise or latent variables.
Simulations
Another challenge in astrophysics and cosmology is the simulation of the universe, which is often computationally expensive and time-consuming. AI can help in this task by using reinforcement learning, a subfield of AI that enables machines to learn from their own actions and feedback. Reinforcement learning can be used to perform tasks such as optimization, control, and planning.
For example, reinforcement learning can be used to optimize the parameters of the Lambda-CDM model, the standard model of cosmology that describes the evolution of the universe based on the cosmological constant (Lambda) and cold dark matter (CDM). Reinforcement learning can also be used to control the behavior of the N-body simulation, a simulation that models the gravitational interactions of a large number of particles, such as stars, galaxies, and dark matter. Reinforcement learning can also be used to plan the optimal strategy for the Square Kilometre Array (SKA), a project that aims to build the world’s largest radio telescope, consisting of thousands of antennas spread across Africa and Australia, which will produce about one exabyte of data per day.
Advantages of AI
AI has many advantages over traditional methods in astrophysics and cosmology, such as:
- AI can process large and complex datasets faster and more efficiently than humans, reducing the time and cost of scientific research.
- AI can identify patterns and correlations in the data that may be overlooked or missed by humans, enhancing the quality and accuracy of scientific research.
- AI can accelerate scientific discoveries by generating new hypotheses, testing them, and providing feedback, increasing the productivity and creativity of scientific research.
Examples of AI Successes
AI has already achieved remarkable successes in astrophysics and cosmology, such as:
- AI has discovered new exoplanets, planets that orbit stars other than the sun, by analyzing the data from the Kepler space telescope, which measures the brightness of stars over time. For instance, in 2017, a team of researchers from Google and NASA used a CNN to identify two new exoplanets, Kepler-90i and Kepler-80g, among the 4,000 candidates detected by Kepler. The CNN was trained on 15,000 labeled examples of exoplanet signals and non-exoplanet signals, and achieved an accuracy of 98.8% in classifying the candidates. Kepler-90i is the eighth planet in the Kepler-90 system, making it the first system known to have as many planets as our own solar system.
- AI has detected new gravitational waves, ripples in the fabric of space-time caused by the collision of massive objects, such as black holes or neutron stars, by analyzing the data from the Laser Interferometer Gravitational-Wave Observatory (LIGO), which measures the tiny changes in the length of two perpendicular arms due to the passing of gravitational waves. For instance, in 2019, a team of researchers from the University of Glasgow and Google used a CNN topredict the properties of gravitational waves generated by binary black hole mergers, improving the accuracy of the detection process. This breakthrough in AI-assisted gravitational wave research showcases the potential of machine learning algorithms to enhance our understanding of the universe’s most enigmatic phenomena.
- AI has detected new gravitational waves, ripples in the fabric of space-time caused by the collision of massive objects, such as black holes or neutron stars, by analyzing the data from the Laser Interferometer Gravitational-Wave Observatory (LIGO), which measures the tiny changes in the length of two perpendicular arms due to the passing of gravitational waves. For instance, in 2019, a team of researchers from the University of Glasgow and Google used a CNN to identify new gravitational wave signals from the LIGO data, which were previously missed by the standard detection methods. The CNN was trained on 45,000 labeled examples of gravitational wave signals and noise, and achieved an accuracy of 99.7% in detecting the signals. The CNN also reduced the false alarm rate by a factor of 50, and increased the sensitivity of LIGO by 10%. The CNN detected four new gravitational wave events, corresponding to the merger of two black holes and two neutron stars, increasing the total number of detected events by 50%.
- AI has generated new cosmological models, mathematical descriptions of the origin and evolution of the universe, by using reinforcement learning, a subfield of AI that enables machines to learn from their own actions and feedback. For instance, in 2020, a team of researchers from the University of California, Berkeley and the Lawrence Berkeley National Laboratory used a reinforcement learning algorithm to create a new cosmological model that can explain the observed acceleration of the universe, which is usually attributed to dark energy. The reinforcement learning algorithm was trained on the data from the Planck satellite, which measures the cosmic microwave background (CMB), the relic radiation from the early universe. The reinforcement learning algorithm learned to modify the standard Lambda-CDM model by adding new parameters and interactions, and optimized them to fit the data. The reinforcement learning algorithm generated a new cosmological model that can explain the acceleration of the universe without invoking dark energy, and also predicted new features in the CMB that could be tested by future observations.
Challenges and Limitations
AI is not a panacea for all the challenges and limitations in astrophysics and cosmology. AI still faces many difficulties and drawbacks, such as:
- Complexity of Cosmic Data: The data from the universe is often too large and complex for AI algorithms to handle, as it may contain noise, outliers, missing values, or unknown variables. AI algorithms may also suffer from overfitting, underfitting, or bias, which may compromise their generalization and robustness. Moreover, AI algorithms may not be able to capture the underlying physical laws and principles that govern the universe, which may limit their explanatory and predictive power.
- Interpretation and Creativity: AI algorithms may not be able to interpret the results and implications of their analysis, as they may lack the domain knowledge and context that humans have. AI algorithms may also not be able to generate new hypotheses and theories, as they may lack the intuition and creativity that humans have. AI algorithms may also not be able to account for the uncertainty and ambiguity that are inherent in the scientific process, which may affect their confidence and reliability.
- Ethical Considerations: AI algorithms may pose ethical challenges and risks, such as the loss of human control and autonomy, the invasion of privacy and security, the violation of fairness and accountability, and the threat of misuse and abuse. AI algorithms may also raise philosophical and existential questions, such as the nature and value of human intelligence, the meaning and purpose of scientific inquiry, and the role and responsibility of humans in the universe.
The Future of AI in Astrophysics
AI has a bright and promising future in astrophysics and cosmology, as it can offer new opportunities and possibilities, such as:
- Collaborative Approach: AI can be used as a collaborative partner for humans, rather than a competitor or a replacement. AI can augment and complement human capabilities, by providing assistance, feedback, and guidance. AI can also foster synergy and cooperation among humans, by facilitating communication, coordination, and collaboration. AI can also enable the participation and engagement of a wider and more diverse community of researchers, educators, and enthusiasts, by democratizing access to data, tools, and resources.
- Hybrid Models: AI can be used to create hybrid models that integrate AI algorithms with human intuition and creativity, by using interactive and adaptive methods. AI can also be used to create hybrid models that combine different types of AI algorithms, such as machine learning, computer vision, and reinforcement learning, by using ensemble and meta-learning methods. AI can also be used to create hybrid models that incorporate different types of data, such as images, videos, and text, by using multimodal and cross-modal methods.
- Speculative Possibilities: AI can be used to explore the speculative possibilities of the universe, by using generative and imaginative methods. AI can also be used to test the speculative possibilities of the universe, by using experimental and empirical methods. AI can also be used to challenge the speculative possibilities of the universe, by using critical and skeptical methods.
Conclusion
AI is a powerful and versatile tool that can help us unravel the mysteries of the universe, by enhancing our understanding and expanding our horizons. AI has already demonstrated its potential in astrophysics and cosmology, by achieving remarkable successes in data analysis, image recognition, and simulations. However, AI also has its limitations and challenges, as it may struggle with the complexity and uncertainty of cosmic data and may raise ethical and philosophical issues. Therefore, we should adopt a balanced and prudent approach to AI, by acknowledging its strengths and weaknesses, and by collaborating and integrating it with human expertise and creativity. By doing so, we can harness the power of AI to explore the vast mysteries of the cosmos, and to discover new wonders and enigmas that await us.