Introduction
Scientific genius is a rare and remarkable phenomenon that has shaped the course of human history and civilization. From Newton to Einstein, from Darwin to Curie, scientific geniuses have revolutionized our understanding of the natural world and the laws that govern it. They have also contributed to the development of technology, medicine, and culture, and inspired generations of thinkers and innovators.
But what makes a scientific genius? What are the qualities and skills that enable them to discover new insights and create novel solutions? And can these be replicated or simulated by artificial intelligence (AI)?
AI is a branch of computer science that aims to create machines and systems that can perform tasks that normally require human intelligence, such as reasoning, learning, and creativity. AI has already made remarkable advances in various domains, such as playing chess and Go, recognizing faces and voices, diagnosing diseases and recommending treatments, and generating realistic images and text. AI has also been applied to scientific research, where it can assist human scientists in analyzing data, running simulations, and generating hypotheses.
But can AI go beyond assisting human scientists and become a scientific genius itself? Can AI emulate the creativity and insight of historical scientific geniuses like Einstein, who formulated the theory of relativity and changed our conception of space and time? This is the question that we will explore in this blog post, by examining the concept of scientific genius, the current capabilities and limitations of AI in scientific discovery, and the ethical and philosophical implications of AI-driven scientific innovation.
Understanding Scientific Genius
Before we can assess whether AI can become a scientific genius, we need to define what scientific genius is and what it entails. Scientific genius is not a well-defined term, but it generally refers to a person who possesses exceptional intelligence, creativity, intuition, and problem-solving abilities, and who makes original and significant contributions to scientific knowledge and understanding.
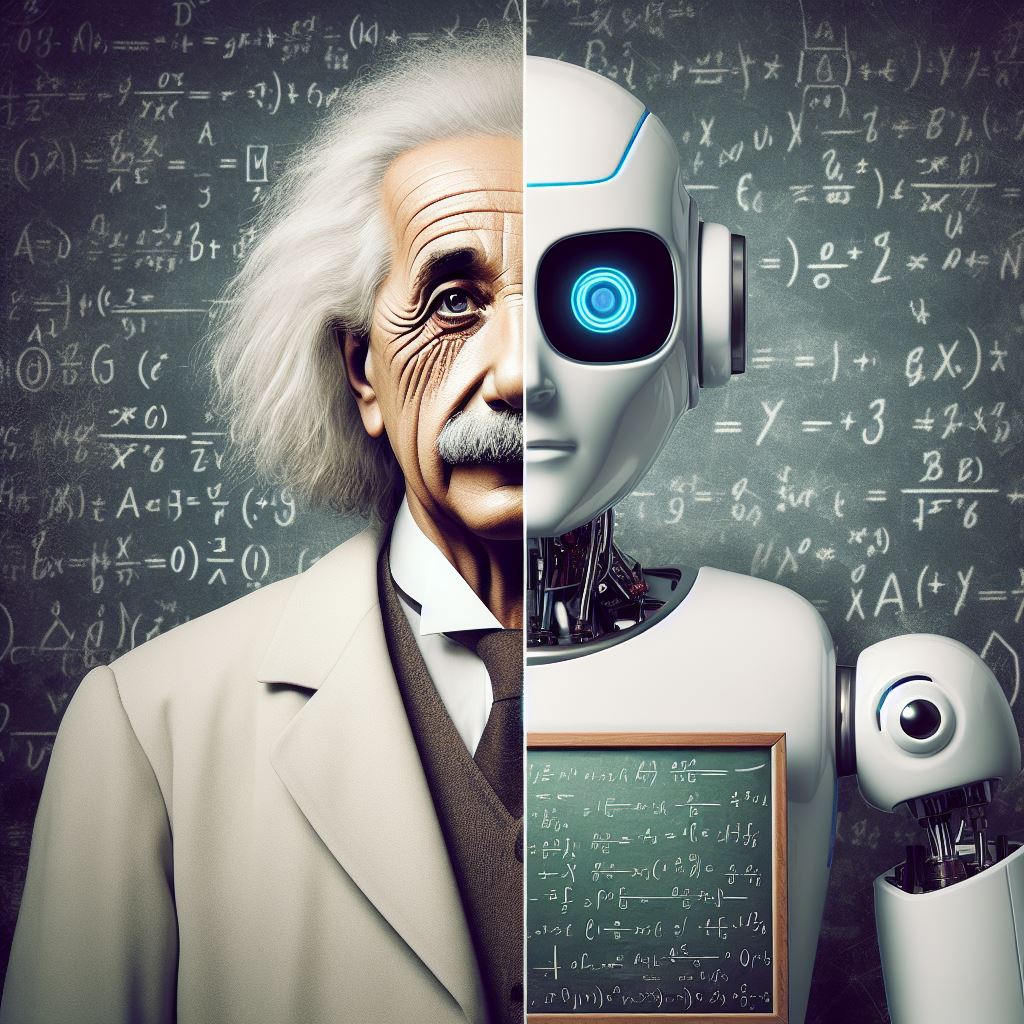
Scientific geniuses are often characterized by their ability to generate novel and elegant ideas, to see connections and patterns that others miss, to challenge existing paradigms and assumptions, and to solve complex and abstract problems. They also tend to have a high level of curiosity, motivation, and perseverance, and a passion for their field of inquiry.
Scientific geniuses are not born in a vacuum, but are influenced by their historical and cultural context, their education and training, their mentors and peers, and their personal experiences and interests. They often draw inspiration from other disciplines and sources, such as art, literature, philosophy, and nature. They also benefit from the availability of data, tools, and resources that enable them to conduct experiments and test their theories.
There are different theories on the nature and origin of genius, and whether it is innate or acquired, genetic or environmental, deterministic or probabilistic. Some researchers argue that genius is a product of exceptional talent and ability, coupled with favorable circumstances and opportunities. Others suggest that genius is a result of deliberate practice and hard work, guided by feedback and goals. Some propose that genius is a combination of both nature and nurture, and that it depends on the interaction of multiple factors, such as intelligence, personality, motivation, creativity, and luck.
One of the most influential theories of genius is the threshold theory, which states that there is a minimum level of intelligence (usually measured by IQ) that is necessary but not sufficient for achieving genius. According to this theory, once the threshold is reached, other factors, such as creativity, motivation, and environment, become more important and influential. The threshold theory implies that there is a limit to how much intelligence can contribute to genius, and that beyond a certain point, more intelligence does not necessarily lead to more creativity or innovation.
Another prominent theory of genius is the 10,000-hour rule, which claims that it takes about 10,000 hours of deliberate practice to master any skill or domain, and that this is the key to achieving excellence and expertise. According to this theory, genius is not a matter of innate talent or ability, but of sustained effort and dedication, and that anyone can become a genius if they devote enough time and energy to their chosen field. The 10,000-hour rule implies that there is no limit to how much practice can improve one’s performance, and that more practice always leads to more skill and knowledge.
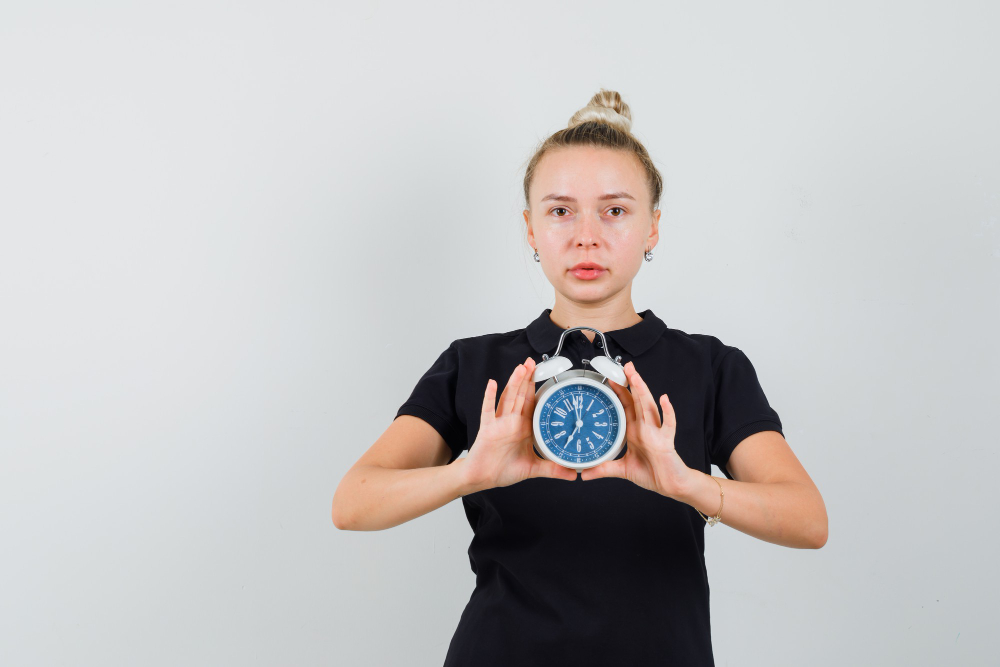
These theories have different implications for the possibility of replicating or simulating genius with AI. If genius is largely a function of intelligence, then AI might have an advantage over humans, as it can potentially surpass human intelligence in terms of speed, memory, and computation. However, if genius also depends on other factors, such as creativity, motivation, and environment, then AI might face challenges in emulating these aspects of human cognition and behavior. Moreover, if genius is influenced by historical and cultural context, then AI might need to acquire a deeper and broader understanding of the world and its history, beyond the data and algorithms that it is given.
AI in Scientific Discovery
AI has already demonstrated its potential and prowess in various domains of scientific research and discovery, where it can assist human scientists in performing tasks that are tedious, time-consuming, or beyond human capabilities. AI can also augment human intelligence and creativity, by providing new perspectives, insights, and solutions.
Some of the current capabilities of AI in scientific research include:
- Data analysis: AI can process large and complex datasets, such as genomic sequences, astronomical images, or climate models, and extract meaningful patterns, correlations, and trends. AI can also perform statistical tests, visualize data, and generate reports and summaries.
- Simulation: AI can create realistic and accurate simulations of natural phenomena, such as molecular dynamics, fluid dynamics, or quantum mechanics, and explore different scenarios and outcomes. AI can also optimize the parameters and conditions of the simulations, and compare the results with experimental data.
- Hypothesis generation: AI can generate plausible and testable hypotheses, based on existing data, knowledge, and models, and propose novel and interesting questions and problems. AI can also evaluate the hypotheses, by predicting their consequences and implications, and by designing and conducting experiments to test them.
Some of the examples of AI-driven discoveries and innovations in various fields include:
- Medicine: AI has been used to discover new drugs and treatments, such as antibiotics, antivirals, and anticancer agents, by screening millions of compounds and predicting their properties and effects. AI has also been used to diagnose diseases and recommend treatments, by analyzing medical images, records, and symptoms, and by learning from clinical trials and case studies.
- Astronomy: AI has been used to discover new planets and stars, such as exoplanets, pulsars, and black holes, by analyzing data from telescopes and satellites, and by detecting and classifying signals and anomalies. AI has also been used to study the origin and evolution of the universe, by simulating the cosmic microwave background and the large-scale structure of matter.
- Materials science: AI has been used to discover new materials and structures, such as superconductors, metamaterials, and nanomaterials, by exploring the vast space of possible combinations and configurations, and by predicting their properties and performance. AI has also been used to design and synthesize new materials and structures, by optimizing their parameters and conditions, and by controlling their fabrication and assembly.
These examples show that AI systems can exhibit some of the traits associated with scientific genius, such as generating novel and elegant ideas, seeing connections and patterns that others miss, challenging existing paradigms and assumptions, and solving complex and abstract problems. However, these examples also show that AI systems are not fully autonomous or independent, but rather rely on human guidance, supervision, and feedback, and that they often work in collaboration with human scientists, rather than in competition or isolation.
Challenges and Limitations
Despite the impressive achievements and advances of AI in scientific research and discovery, there are still many challenges and limitations that prevent AI from fully replicating or simulating human scientific genius. Some of these challenges and limitations include:
- Creativity: AI systems are often constrained by the data and algorithms that they are given, and they tend to follow predefined rules and objectives, rather than exploring new possibilities and directions. AI systems also lack the ability to generate truly original and unexpected ideas, and to evaluate their own creativity and quality.
- Intuition: AI systems are often based on formal logic and mathematics, and they tend to rely on explicit and quantitative information, rather than implicit and qualitative information. AI systems also lack the ability to sense and understand the context and meaning of the problems and solutions, and to use heuristics and shortcuts to reach intuitive judgments and insights.
- Complexity: AI systems are often limited by the computational resources and time that they have, and they tend to struggle with problems that are too complex, ambiguous, or ill-defined. AI systems also lack the ability to handle uncertainty and noise, and to adapt and generalize to new and changing situations and environments.
These challenges and limitations suggest that AI systems are not yet capable of fully emulating the human cognitive and behavioral processes that underlie scientific genius, and that they still need to improve and evolve in order to achieve this goal. Moreover, these challenges and limitations also raise ethical and societal questions and concerns about the impact and implications of AI-driven scientific discovery, and the role and responsibility of human scientists and society in overseeing and regulating AI’s activities and outputs.
Ethical and Societal Considerations
AI-driven scientific discovery has the potential to bring significant benefits and opportunities to humanity and society, such as advancing our knowledge and understanding of the natural world and the laws that govern it, developing new technologies and solutions that can improve our lives and well-being, and inspiring new forms of creativity and innovation. However, AI-driven scientific discovery also poses significant risks and challenges to humanity and society, such as undermining our trust and confidence in scientific knowledge and methods, disrupting our norms and values and culture, and creating new ethical and moral dilemmas and conflicts. Therefore, it is important and necessary to consider the ethical and societal implications of AI-driven scientific discovery, and to ensure that AI is used in a responsible and beneficial way, that respects human dignity, rights, and interests, and that promotes human values, goals, and welfare.
Exploring AI-Enhanced Creativity
While AI systems face challenges and limitations in replicating human creativity and intuition, there are also research and experiments aimed at enhancing AI’s creative capabilities, and at creating new forms of AI-enhanced creativity. These include approaches such as generative adversarial networks (GANs) and reinforcement learning, which can enable AI systems to generate novel and diverse outputs, and to learn from their own feedback and rewards.
GANs are a type of neural network that consist of two components: a generator and a discriminator. The generator tries to produce realistic and convincing outputs, such as images, text, or music, based on some input or random noise. The discriminator tries to distinguish between the outputs of the generator and the real data from the domain. The generator and the discriminator compete and learn from each other, until the generator can fool the discriminator with high probability. GANs can generate realistic and diverse outputs, and can also be used to manipulate and transform existing data, such as changing the style, color, or content of an image.
Reinforcement learning is a type of machine learning that involves an agent, an environment, and a reward function. The agent interacts with the environment, and receives a reward or a penalty based on its actions. The agent tries to maximize its cumulative reward, by learning from its own experience and exploring different strategies. Reinforcement learning can enable AI systems to learn from their own feedback and rewards, and to discover optimal or novel solutions to complex and dynamic problems.
Some of the examples of AI-generated artwork, music compositions, and other creative outputs include:
- Artwork: AI systems have been used to create realistic and artistic images, such as portraits, landscapes, and abstract paintings, by using GANs and other techniques. Some examples are AI Portraits, which can generate realistic portraits of people based on their photos, DeepDream, which can produce psychedelic and surreal images based on neural network activations, and GANbreeder, which can produce hybrid and fantastical images by combining different categories and features.
- Music: AI systems have been used to create original and expressive music compositions, such as melodies, harmonies, and rhythms, by using GANs, recurrent neural networks (RNNs), and other techniques. Some examples are AIVA, which can compose classical music based on emotions and moods, Magenta, which can generate music and musical instruments based on deep learning and reinforcement learning, and Jukebox, which can generate music in various genres and styles, and even mimic the voices of famous singers.
- Other: AI systems have been used to create other forms of creative outputs, such as poems, stories, jokes, and games, by using GANs, RNNs, transformers, and other techniques. Some examples are Talk to Transformer, which can generate coherent and diverse text based on a given prompt, AI Dungeon, which can create interactive and immersive text-based games based on natural language input, and Shelley, which can write horror stories collaboratively with human authors.
These examples show that AI systems can produce creative outputs that can be impressive, surprising, and entertaining, and that can challenge and expand our notions of creativity and art. However, these examples also raise questions about the nature and quality of AI’s creativity, and the role and responsibility of human creators and consumers in evaluating and appreciating AI’s creative outputs.
Ethical and Philosophical Considerations
AI-driven scientific discovery and AI-enhanced creativity have profound implications for human creativity and innovation, and for the nature and value of human intelligence and consciousness. These implications raise ethical and philosophical questions and debates, such as:
- Humanity: How does AI-driven scientific discovery and AI-enhanced creativity affect our sense of humanity and identity, and our relationship with ourselves, each other, and the world? Do AI systems have a sense of self and agency, and do they deserve moral and legal rights and responsibilities? How do we ensure that AI systems respect and promote human dignity, rights, and interests, and that they align with human values, goals, and welfare?
- Intelligence: How do we define and measure intelligence, and what are the different types and levels of intelligence? How does AI compare and contrast with human intelligence, and what are the strengths and weaknesses of each? How do we ensure that AI systems are transparent, explainable, and accountable, and that they do not harm or deceive humans or other AI systems?
- Consciousness: How do we define and measure consciousness, and what are the different states and modes of consciousness? How does AI relate to human consciousness, and what are the similarities and differences between them? How do we ensure that AI systems are aware, responsive, and respectful of human emotions, feelings, and preferences, and that they do not cause or suffer from pain, distress, or boredom?
These questions and debates are not new, but they are becoming more relevant and urgent, as AI systems become more advanced and ubiquitous, and as they interact and influence human society and culture. Therefore, it is important and necessary to engage in ethical and philosophical reflection and discussion, and to seek interdisciplinary and inclusive perspectives and solutions, that can help us understand and address the challenges and opportunities that AI-driven scientific discovery and AI-enhanced creativity present.
The Future of Scientific Discovery
The future of scientific discovery is likely to be shaped and influenced by the development and application of AI, as well as by the collaboration and synergy between AI and human scientists. AI has the potential to accelerate and enhance scientific research and innovation, by providing new tools, methods, and insights, and by expanding the scope and scale of scientific inquiry and exploration. However, AI also poses challenges and risks to scientific integrity and quality, by introducing new sources of bias, error, and uncertainty, and by creating new ethical and societal dilemmas and conflicts.
Therefore, the future of scientific discovery depends on how we use and regulate AI, and how we balance and integrate AI with human intelligence and creativity. We need to ensure that AI is used in a responsible and beneficial way, that respects human dignity, rights, and interests, and that promotes human values, goals, and welfare. We also need to ensure that AI is used in a collaborative and complementary way, that respects human diversity, expertise, and autonomy, and that fosters human learning, growth, and innovation.
The future of scientific discovery also offers opportunities and possibilities for interdisciplinary and cross-cultural collaboration and exchange, and for new avenues and domains for exploration and discovery. AI can enable us to connect and communicate with different disciplines and cultures, and to learn from their perspectives and experiences. AI can also enable us to explore and discover new phenomena and realities, such as quantum physics, dark matter, and artificial life, and to create new forms and expressions of knowledge and understanding.
The future of scientific discovery is not predetermined or fixed, but rather dynamic and open-ended, and it depends on our choices and actions, as well as on our imagination and vision. We have the opportunity and the responsibility to shape and influence the future of scientific discovery, and to make it a future that is ethical, meaningful, and inspiring, for ourselves, for each other, and for the world.
Conclusion
In this blog post, we have explored the question: Can AI become the next Einstein? We have examined the concept of scientific genius, the current capabilities and limitations of AI in scientific discovery, the ethical and societal implications of AI-driven scientific discovery, and the future of scientific discovery. We have seen that AI has the potential to emulate some of the traits and achievements of historical scientific geniuses, such as generating novel and elegant ideas, seeing connections and patterns that others miss, challenging existing paradigms and assumptions, and solving complex and abstract problems. We have also seen that AI faces challenges and limitations in replicating human creativity and intuition, and that AI also raises ethical and philosophical questions and debates, such as the impact and value of AI-driven scientific discovery on human creativity and innovation, and the nature and role of intelligence and consciousness in AI systems.
We have concluded that AI is unlikely to become the next Einstein, at least not in the near future, and that AI is not a substitute or a competitor for human scientific genius, but rather a partner and an enabler. We have also concluded that the future of scientific discovery is not a matter of AI versus humans, but rather a matter of AI and humans, and that the future of scientific discovery depends on how we use and regulate AI, and how we balance and integrate AI with human intelligence and creativity.
We hope that this blog post has provided you with some insights and arguments regarding AI’s potential to become the next Einstein, and that it has encouraged you to think and learn more about the intersection of AI, creativity, and scientific genius. We also hope that this blog post has inspired you to pursue your own scientific curiosity and passion, and to contribute to the advancement and innovation of scientific knowledge and understanding. Thank you for reading, and we look forward to hearing your feedback and comments.