Introduction
In recent years, artificial intelligence (AI) has made significant strides in transforming various industries, and healthcare is no exception. One of the most promising advancements is the integration of AI-driven diagnostic tools, which are revolutionizing patient care by enhancing the accuracy and speed of disease detection. These tools utilize complex algorithms and vast amounts of data to assist healthcare professionals in making more informed decisions, ultimately improving patient outcomes.
Traditional diagnostic methods, while effective, often come with limitations such as human error, time constraints, and the need for extensive resources. AI-driven diagnostics address these challenges by offering more precise and rapid analysis, reducing the margin for error, and enabling early detection of diseases. From analyzing medical images to predicting disease outbreaks, AI is poised to play a pivotal role in the future of healthcare.
The Evolution of Diagnostic Tools
Diagnostic tools have come a long way from their rudimentary beginnings. Historically, physicians relied on basic techniques such as visual inspection, palpation, and rudimentary laboratory tests to diagnose ailments. The invention of the microscope in the 17th century marked a significant leap, allowing for the observation of microorganisms and cells, which paved the way for more accurate diagnostics.
The late 20th century saw the introduction of digital diagnostics, significantly enhancing the precision and scope of medical testing. Technologies such as magnetic resonance imaging (MRI), computed tomography (CT) scans, and ultrasound revolutionized how doctors diagnose and monitor diseases. However, these methods still required significant human interpretation, which could lead to variability and error.
Enter the 21st century, where artificial intelligence began to make its mark. AI-driven diagnostic tools leverage machine learning algorithms to analyze vast datasets, identifying patterns and anomalies with a level of precision and speed unattainable by human analysis alone. For instance, AI can now interpret medical images with remarkable accuracy, often surpassing the diagnostic capabilities of human radiologists (AI-Driven Healthcare Diagnostics, AHA).
Early applications of AI in healthcare have shown promising results. For example, DeepMind’s AI technology has been utilized to reduce MRI scanning time by 90%, enhancing image quality by minimizing patient movement artifacts. Similarly, AI systems like Lunit’s have been used to detect lung nodules from chest X-rays and CT scans, significantly improving diagnostic accuracy (AI-Driven Healthcare Diagnostics, GlobalSpec).
These advancements are not limited to imaging. AI algorithms are also being employed to analyze patient records, using natural language processing to extract meaningful insights from unstructured data such as doctor’s notes and patient histories. This not only aids in quicker diagnosis but also helps in creating comprehensive patient profiles, paving the way for personalized medicine (AHA, GlobalSpec).
How AI-Driven Diagnostic Tools Work
Artificial intelligence (AI) refers to the simulation of human intelligence in machines that are programmed to think and learn like humans. Machine learning (ML), a subset of AI, involves the use of algorithms and statistical models to enable machines to improve their performance on tasks through experience. Essentially, these technologies allow computers to analyze data, recognize patterns, and make decisions with minimal human intervention.
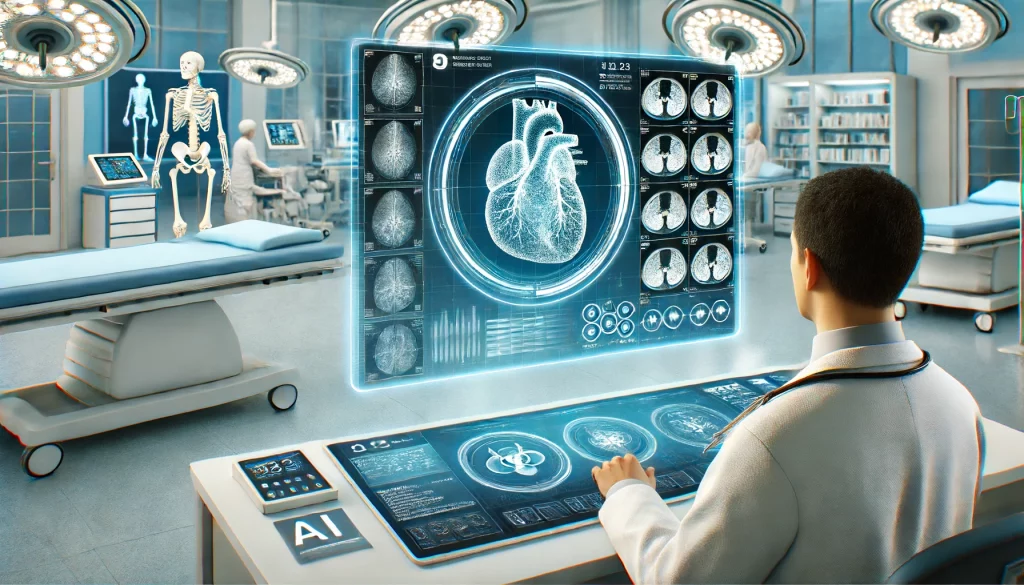
In the context of healthcare, AI and ML are used to process large amounts of medical data, ranging from images and genetic information to electronic health records. By identifying patterns and correlations within this data, AI can assist in diagnosing diseases, predicting patient outcomes, and recommending treatments.
AI-driven diagnostic tools rely on vast datasets to function effectively. These datasets can include medical images (such as X-rays, CT scans, and MRIs), patient records, laboratory test results, and even real-time data from wearable devices. The data collection process involves gathering, digitizing, and standardizing this information to ensure consistency and accuracy.
Once the data is collected, it undergoes preprocessing, which includes steps like normalization, anonymization, and augmentation to prepare it for analysis. Machine learning models are then trained on this data, allowing the AI to learn from examples. For instance, a model might be trained on thousands of labeled medical images to learn how to identify signs of specific diseases (AI-Driven Healthcare Diagnostics, AHA, GlobalSpec).
Several key technologies underpin AI-driven diagnostic tools. Neural networks are a type of machine learning model inspired by the human brain’s structure and function. They are particularly effective for image and speech recognition tasks. Natural language processing (NLP) enables machines to understand and interpret human language, making it possible to analyze unstructured data like doctor’s notes and medical literature. Deep learning, a subset of machine learning that uses neural networks with many layers, is especially powerful for processing complex data such as medical images and genomic sequences.
Benefits of AI in Diagnostics
AI-driven diagnostic tools offer several significant benefits. Firstly, they improve accuracy and enable early detection of diseases. By analyzing vast amounts of data and identifying patterns that might be missed by human eyes, AI can detect conditions at an earlier stage, which is crucial for effective treatment. For instance, AI algorithms have shown exceptional accuracy in diagnosing diseases like cancer, often identifying malignancies in medical images with higher precision than human radiologists (AI-Driven Healthcare Diagnostics, AHA).
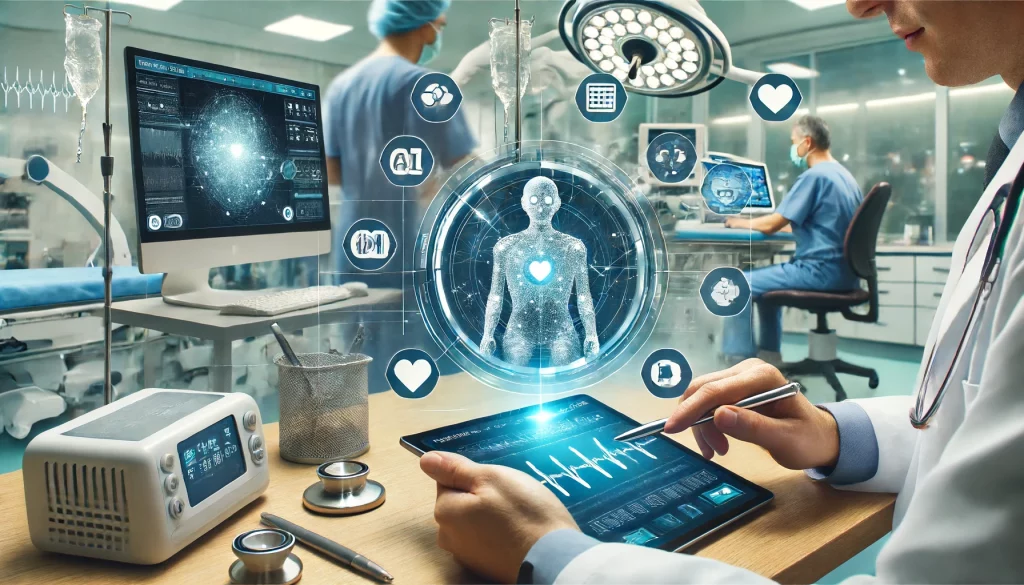
Moreover, AI reduces the potential for human error, which is a significant concern in medical diagnostics. Fatigue, oversight, and subjective judgment can lead to mistakes, but AI systems can provide consistent and objective analysis. This consistency helps in standardizing diagnostic procedures and ensuring that patients receive accurate and timely diagnoses.
Another key benefit is the efficiency and speed of AI diagnostics. Traditional diagnostic methods can be time-consuming and resource-intensive, often requiring multiple tests and consultations. AI-driven tools can streamline this process by quickly analyzing data and providing diagnostic results in a fraction of the time. This not only enhances patient care but also reduces the workload on healthcare professionals, allowing them to focus more on patient interaction and treatment planning (GlobalSpec).
Cost-effectiveness is another advantage. Although the initial investment in AI technology can be substantial, the long-term savings are significant. By reducing the need for repeated tests and minimizing errors, AI diagnostics can lower healthcare costs and make medical services more accessible to a broader population.
Applications in Various Medical Fields
The applications of AI-driven diagnostic tools are vast and varied across different medical fields. In radiology, AI is used to analyze medical images, such as X-rays, CT scans, and MRIs. AI algorithms can detect abnormalities, measure organ sizes, and even predict the likelihood of diseases such as cancer. For instance, AI systems have been developed to identify lung nodules in chest X-rays, assisting radiologists in diagnosing lung cancer at an early stage (AHA, AI-Driven Healthcare Diagnostics).
In pathology, AI-driven tools can analyze tissue samples and detect patterns indicative of diseases. These tools can identify cancerous cells in biopsy samples, helping pathologists make more accurate diagnoses. Similarly, in cardiology, AI is used to interpret ECGs and identify heart conditions such as arrhythmias and heart failure.
Oncology is another field where AI is making significant contributions. AI algorithms can analyze genetic data to identify mutations associated with different types of cancer. This information is crucial for developing personalized treatment plans tailored to the specific genetic makeup of the patient’s cancer, leading to more effective therapies and better patient outcomes (GlobalSpec).
Challenges and Ethical Considerations
Despite the numerous benefits, the integration of AI in diagnostics also presents several challenges and ethical considerations. One major concern is privacy and data security. AI systems rely on vast amounts of patient data, which must be protected to prevent breaches and unauthorized access. Ensuring the privacy of patient information is paramount, and robust cybersecurity measures must be in place to safeguard this data (Revolutionizing Healthcare: The Role of Artificial Intelligence in Clinical Practice).
Ethical considerations also include the need for transparency and accountability in AI decision-making. It is essential for AI systems to be transparent about how they arrive at their conclusions, and there must be accountability for any errors or biases that may arise. Addressing algorithmic bias is critical to ensuring that AI systems provide fair and equitable diagnoses, especially for vulnerable and underrepresented populations.
Regulatory frameworks are needed to guide the development and deployment of AI in healthcare. These frameworks should ensure that AI systems meet high standards of safety, efficacy, and ethical conduct. Collaboration between healthcare providers, AI developers, and regulatory bodies is essential to creating a robust and trustworthy AI-driven diagnostic ecosystem. This will help in ensuring that AI technologies are deployed in ways that maximize their benefits while minimizing potential risks.
The Future of AI in Healthcare
As AI continues to evolve, its potential to transform healthcare grows. Future advancements may include more sophisticated algorithms capable of diagnosing a wider range of diseases with even greater accuracy. Integration of AI with other emerging technologies, such as genomics and personalized medicine, will likely lead to more individualized and effective treatment plans.
AI-driven diagnostic tools are also expected to become more accessible, potentially being deployed in remote and underserved areas where healthcare resources are limited. This could democratize access to high-quality diagnostics, reducing health disparities and improving global health outcomes.
In conclusion, AI is revolutionizing the field of diagnostics, offering unprecedented accuracy, efficiency, and cost-effectiveness. While there are challenges to overcome, the benefits of AI-driven diagnostics are undeniable. As we continue to navigate the ethical and regulatory landscape, it is crucial to ensure that these powerful tools are used responsibly to enhance patient care and improve health outcomes worldwide.
FAQs on AI-Driven Diagnostic Tools in Healthcare
1. How does AI improve the accuracy of medical diagnoses?
AI improves the accuracy of medical diagnoses by analyzing large datasets and identifying patterns that may be missed by human eyes. Machine learning algorithms are trained on vast amounts of medical data, allowing them to detect diseases at early stages with high precision. AI’s ability to process and analyze data quickly and accurately reduces the margin of error and enhances the reliability of diagnostic results.
2. What are the main challenges in implementing AI-driven diagnostic tools in healthcare?
The main challenges include ensuring data privacy and security, addressing ethical considerations such as algorithmic bias, and establishing regulatory frameworks to ensure safety and efficacy. AI systems require vast amounts of patient data, which must be protected from breaches and unauthorized access. Transparency in AI decision-making and accountability for errors are also critical to gaining trust in these technologies.
3. How can AI-driven diagnostic tools be used in remote and underserved areas?
AI-driven diagnostic tools can be deployed in remote and underserved areas through telemedicine platforms and portable diagnostic devices. These tools can analyze medical images, patient records, and other data remotely, providing accurate diagnoses without the need for on-site specialists. This can significantly improve access to high-quality healthcare in regions with limited medical resources, helping to reduce health disparities.
4. What is the role of AI in personalized medicine?
AI plays a crucial role in personalized medicine by analyzing genetic, clinical, and lifestyle data to create tailored treatment plans for individual patients. By identifying specific genetic mutations and patient characteristics, AI can help healthcare providers choose the most effective therapies and interventions. This approach enhances treatment outcomes and minimizes adverse effects, leading to more personalized and precise healthcare.