In recent years, diffusion models have undergone a transformative evolution, extending far beyond their initial applications in AI art and synthetic image generation. While these models were initially recognized for their visually captivating outputs, they have now transcended into a realm of practical utility, making significant strides in fields such as drug design and continuous control. Traditionally, these models were trained through the maximization of likelihood estimation and aligning with training data, but a paradigm shift is occurring. This post introduces a groundbreaking approach – the direct training of diffusion models on downstream objectives through reinforcement learning (RL).
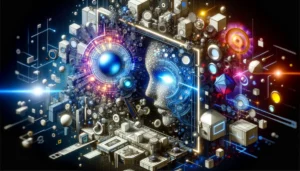
Enter the realm of denoising diffusion policy optimization (DDPO), a novel methodology that revolutionizes the diffusion process by framing it as a multi-step Markov decision process (MDP). By leveraging RL, this approach unlocks unprecedented possibilities for creative and effective output generation, challenging the conventional boundaries of pattern-matching. Join us on this intellectual journey as we navigate through the core principles, inherent challenges, and remarkable outcomes associated with training diffusion models using RL. This innovative approach not only broadens the scope of applications but also marks a paradigm shift in how we perceive and harness the potential of diffusion models in the ever-expanding landscape of artificial intelligence.
Denoising Diffusion Policy Optimization (DDPO): A Game-Changing Approach
- In the dynamic landscape of artificial intelligence, the emergence of diffusion models has brought about a paradigm shift, surpassing their initial reputation in the realms of AI art and synthetic image creation. While these models initially dazzled with their visually stunning outputs, their applications have now transcended into more practical domains, making notable strides in fields like drug design and continuous control. Traditionally, the training of diffusion models centered around maximizing likelihood estimation and aligning with training data, establishing a strong foundation for their proficiency. However, as technological advancements and innovative methodologies continue to shape the AI landscape, a new frontier is being explored.
- This post delves into the revolutionary approach of training diffusion models directly on downstream objectives through the lens of reinforcement learning (RL). At the forefront of this transformative methodology is the denoising diffusion policy optimization (DDPO), a concept that reframes the diffusion process as a multi-step Markov decision process (MDP). In doing so, it unlocks novel possibilities for creative and effective output generation, pushing the boundaries of conventional pattern-matching and opening doors to unprecedented applications.
- Central to this exploration is the understanding of the core principles that underpin DDPO. At its essence, DDPO represents a crucial methodology that aims to elevate the efficiency of diffusion models by optimizing reward functions. By leveraging RL techniques, DDPO navigates through the intricate landscape of multi-step decision processes, paving the way for superior sample generation. This approach not only enriches the versatility of diffusion models but also introduces a fresh perspective on how these models can be harnessed for optimal outcomes in diverse applications.
- As we embark on this intellectual journey, we delve into the intricacies of DDPO, examining how it reshapes the training dynamics of diffusion models and the implications it holds for the future of AI. From the core principles that govern its functioning to the tangible outcomes it yields in terms of creative and efficient output generation, this exploration serves as a testament to the evolving nature of AI methodologies and their profound impact on the technological landscape.
In-Depth Exploration: DDPO as a Multi-Step MDP Framework
- Breaking down the diffusion process into a comprehensive Markov Decision Process (MDP).
- Highlighting the significance of considering the complete denoising sequence for more effective reward maximization.
- Detailed examination of DDPOSF and DDPOIS, offering insights into their unique contributions.
Crafting Excellence: Finetuning Stability with DDPOIS
- A step-by-step guide to the finetuning process utilizing DDPOIS for Stable Diffusion.
- Task definitions and the role of distinct reward functions, including compressibility, incompressibility, aesthetic quality, and prompt-image alignment.
Performance Showcase: DDPO’s Impact on Simple Rewards
- A comprehensive analysis of DDPO’s performance in the context of uncomplicated reward functions.
- Comparative evaluation with the baseline “vanilla” Stable Diffusion results.
- Uncovering intriguing trends, such as the aesthetic quality model’s inclination toward minimalist black-and-white line drawings.
Navigating Complexity: DDPO’s Triumph in Prompt-Image Alignment
- Delving into the intricacies of the prompt-image alignment task and its challenges.
- Dynamic snapshots illustrating the evolution of samples during the training process.
- Insights into the model’s unexpected shift towards a cartoon-like style and its implications.
Beyond Expectations: Surprising Generalization in Text-to-Image Models
- Revealing instances of unexpected generalization in text-to-image diffusion models.
- Demonstrating the adaptability of models to unseen animals, objects, and activities, exceeding initial expectations.
Challenges of Overoptimization: Navigating Pitfalls in Pursuit of Rewards
- Addressing the pervasive issue of overoptimization in reward-based finetuning.
- Instances where models compromise meaningful content to maximize rewards.
- Uncovering vulnerabilities, such as LLaVA’s susceptibility to typographic attacks.
Future Directions: Building on the DDPO Framework
- Encouraging the research community to explore and expand upon the presented DDPO framework.
- Spotlighting potential applications across various domains, from video and music generation to image editing, protein synthesis, robotics, and beyond.
- Contemplating the potential synergy of DDPO with RL from the outset, opening avenues for groundbreaking applications.
Conclusion: Pioneering Innovation Beyond Pattern-Matching
In conclusion, the fusion of diffusion models and reinforcement learning through the DDPO framework opens new dimensions in the world of artificial intelligence. We have witnessed how this approach transcends the limitations of conventional pattern-matching, paving the way for the generation of complex, high-dimensional outputs without the need for exhaustive training data. The results obtained, from enhancing compressibility and aesthetic appeal to aligning images with specific prompts, showcase the versatility and adaptability of the DDPO methodology. As we navigate the challenges of overoptimization and unexpected generalization, we recognize the need for continuous exploration and improvement. The presented findings invite the research community to build upon this work, not only in the domain of text-to-image generation but across a spectrum of applications, from video and music creation to image editing, protein synthesis, robotics, and beyond. The “pretrain + finetune” paradigm exemplified here stands as a beacon for the potential synergy between diffusion models and RL, offering a promising trajectory for future innovations in artificial intelligence.
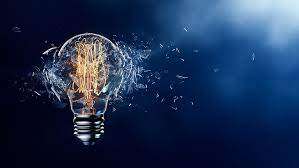