In recent years, diffusion models have undergone a transformative evolution, extending far beyond their initial applications in AI art and synthetic image generation. While these models were initially recognized for their visually captivating outputs, they have now transcended into a realm of practical utility, making significant strides in fields such as drug design and continuous control. Traditionally, these models were trained through the maximization of likelihood estimation and aligning with training data, but a paradigm shift is occurring. This post introduces a groundbreaking approach – the direct training of diffusion models on downstream objectives through reinforcement learning (RL).
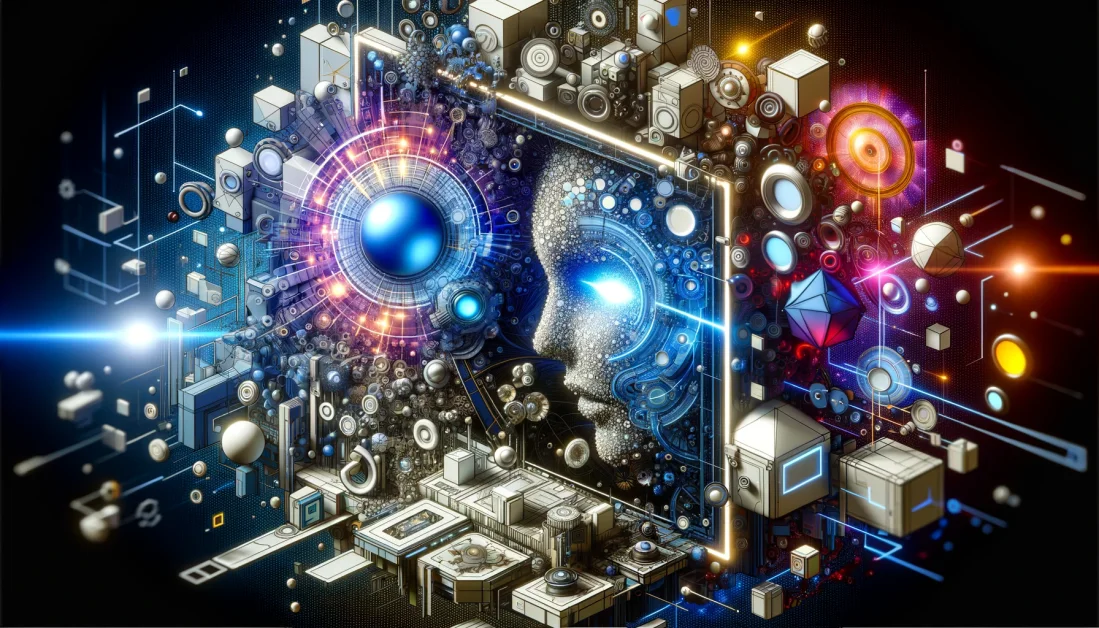