Abstract
We are living in a time of unprecedented change, and the pace of innovation is accelerating at an exponential rate. At the forefront of this transformation is Artificial Intelligence (AI), a technology that has the potential to revolutionize every aspect of our lives. From the simplest tasks to the most complex challenges, AI is poised to transform the fabric of our existence.
As we stand at the threshold of this new era, it’s essential to understand the context and implications of this transformation. AI is not just a technology; it’s a catalyst for change, and its impact will be felt for generations to come. The Age of AI is not just about machines; it’s about us – our relationships, our work, our healthcare, our education, and our very existence.
In this chapter, we’ll embark on a journey to explore the dawn of this new era. We’ll delve into the history of AI, from its early beginnings to the current state of the art. We’ll examine the drivers and enablers of the AI revolution, and we’ll discuss the trends and projections that will shape the future of AI. We’ll also explore the terminology and definitions that are essential to understanding this complex and multifaceted technology.
By the end of this chapter, you’ll have a solid foundation in the fundamentals of AI, and you’ll be ready to dive deeper into the implications and opportunities that this technology presents. So, let’s begin our journey into the Age of AI, and discover the transformative power of this revolutionary technology.
A Brief History of AI
Artificial Intelligence (AI) has a rich and fascinating history that spans several decades. The term “Artificial Intelligence” was first coined in 1956 by computer scientist John McCarthy, but the ideas and concepts that led to the development of AI date back to the 19th century.
In the early days, AI was focused on developing machines that could perform tasks that typically required human intelligence, such as problem-solving, learning, and reasoning. One of the pioneers of AI was Alan Turing, a British mathematician and computer scientist who proposed the Turing Test in 1950. The Turing Test was designed to measure a machine’s ability to exhibit intelligent behavior equivalent to, or indistinguishable from, that of a human.
In the 1950s and 1960s, AI research focused on developing rule-based systems that could reason and solve problems using logical deduction. This approach was led by computer scientists like Allen Newell and Herbert Simon, who developed the first AI program, the Logical Theorist, in 1956.
However, the initial enthusiasm for AI was short-lived, and the field experienced a decline in funding and interest in the 1970s and 1980s. This period, known as the “AI winter,” was due to the limitations of the rule-based approach and the failure to deliver on the promises of AI.
The resurgence of AI in the 1990s and 2000s was driven by advances in machine learning, which enabled machines to learn from data and improve their performance over time. This approach was led by researchers like Yann LeCun, Yoshua Bengio, and Geoffrey Hinton, who developed the backpropagation algorithm and convolutional neural networks (CNNs).
The 21st century has seen an explosion of AI applications, from virtual assistants like Siri and Alexa to self-driving cars and medical diagnosis systems. The availability of large amounts of data, advances in computing power, and the development of deep learning algorithms have all contributed to the current AI revolution.
Today, AI is a global phenomenon, with researchers and developers from around the world working on AI applications that have the potential to transform industries and societies. The history of AI is a testament to the power of human ingenuity and the importance of perseverance in the face of challenges. As we move forward in the Age of AI, it’s essential to understand the roots of this technology and the pioneers who paved the way for the innovations we enjoy today.
Defining AI: Understanding the Terminology
As AI continues to evolve and become more integrated into our daily lives, it’s essential to understand the terminology and definitions that surround this complex and multifaceted technology. Artificial Intelligence (AI) is a broad term that encompasses a range of techniques and approaches used to build machines that can perform tasks that typically require human intelligence.
At its core, AI involves the development of algorithms and models that enable machines to learn, reason, and adapt to new situations. This is achieved through various techniques, including machine learning, deep learning, and natural language processing.
Machine Learning (ML) is a subset of AI that involves training machines on data to enable them to make predictions, classify objects, and make decisions. ML is a key driver of AI’s success, and its applications range from image and speech recognition to recommendation systems and fraud detection.
Deep Learning (DL) is a subset of ML that involves the use of neural networks to analyze data. DL is particularly effective in tasks such as image recognition, natural language processing, and speech recognition.
Natural Language Processing (NLP) is another key area of AI research, focusing on the interaction between humans and machines through language. NLP enables machines to understand, generate, and process human language, with applications in chatbots, virtual assistants, and language translation.
Artificial General Intelligence (AGI) is a term used to describe the hypothetical AI system that possesses the ability to understand, learn, and apply knowledge across a wide range of tasks, similar to human intelligence. AGI is still a topic of debate among researchers, with some arguing that it’s still a distant goal, while others believe it’s a matter of time before we achieve it.
Narrow or Weak AI, on the other hand, refers to AI systems that are designed to perform a specific task, such as facial recognition, language translation, or playing chess. These systems are limited to their programming and data, and do not possess the ability to generalize or adapt to new situations.
Understanding these terms and definitions is crucial for navigating the complex landscape of AI research and applications. As AI continues to evolve and become more integrated into our lives, it’s essential to have a solid grasp of the terminology and concepts that underpin this revolutionary technology.
The AI Revolution: Drivers and Enablers
The AI revolution is a complex and multifaceted phenomenon, driven by a combination of technological, economic, and social factors. Several key drivers and enablers have contributed to the rapid growth and adoption of AI, transforming it from a niche research area to a mainstream technology with far-reaching implications.
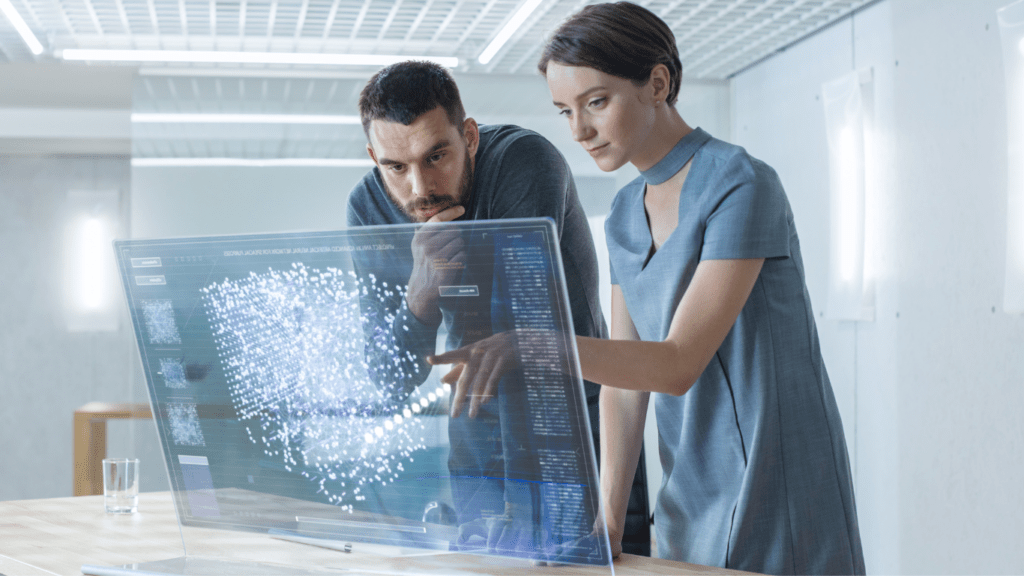
Data Explosion
The exponential growth of data, fueled by the internet, social media, and the Internet of Things (IoT), has created a vast pool of information that can be leveraged by AI algorithms. This data explosion has enabled machines to learn and improve their performance, driving innovation and breakthroughs in areas like image and speech recognition, natural language processing, and predictive analytics.
Advances in Computing Power
The steady increase in computing power, storage capacity, and processing speeds has enabled researchers and developers to build more complex AI models, simulate scenarios, and analyze vast amounts of data. Cloud computing, graphics processing units (GPUs), and tensor processing units (TPUs) have further accelerated AI development, making it possible to train and deploy models at scale.
Algorithmic Innovations
Breakthroughs in machine learning, deep learning, and neural networks have enabled AI systems to learn from data, adapt to new situations, and improve their performance over time. Techniques like transfer learning, generative adversarial networks (GANs), and reinforcement learning have expanded AI’s capabilities, enabling applications like computer vision, natural language processing, and autonomous systems.
Open-Source Contributions
The open-source community has played a crucial role in AI’s development, providing access to libraries, frameworks, and tools like TensorFlow, PyTorch, and scikit-learn. These resources have democratized AI, enabling researchers, developers, and startups to build and deploy AI applications without significant upfront investments.
Investment and Funding
Substantial investments from governments, venture capital firms, and technology giants have fueled AI research and development, enabling the creation of AI-focused startups, research centers, and innovation hubs. This funding has supported the development of AI applications, talent acquisition, and knowledge sharing, driving the AI ecosystem’s growth.
Global Collaboration
The AI revolution is a global phenomenon, with researchers, developers, and innovators collaborating across borders, disciplines, and industries. International conferences, workshops, and research initiatives have facilitated knowledge sharing, idea exchange, and innovation, accelerating AI’s progress and adoption.
These drivers and enablers have collectively contributed to the AI revolution, transforming it from a niche research area to a mainstream technology with far-reaching implications for industries, societies, and individuals. As AI continues to evolve, it’s essential to understand the complex interplay of factors driving its growth and adoption.
The State of AI Today
Artificial Intelligence (AI) has come a long way since its inception, and today it’s a technology that’s transforming industries, societies, and lives. From virtual assistants like Siri, Alexa, and Google Assistant to self-driving cars, medical diagnosis systems, and fraud detection algorithms, AI is everywhere.
Adoption and Applications
AI has been adopted across various industries, including:
- Healthcare: AI-powered diagnosis systems, drug discovery, and personalized medicine
- Finance: Fraud detection, credit scoring, and portfolio management
- Retail: Chatbots, recommendation systems, and predictive analytics
- Manufacturing: Predictive maintenance, quality control, and supply chain optimization
- Transportation: Self-driving cars, traffic management, and route optimization
Success Stories
AI has enabled numerous success stories, such as:
- Google’s AlphaGo defeating a human world champion in Go
- IBM’s Watson winning Jeopardy!
- Amazon’s Alexa becoming a popular virtual assistant
- Waymo’s self-driving cars achieving millions of miles driven
Challenges and Limitations
Despite the progress, AI faces challenges and limitations, including:
- Data quality and bias
- Explainability and transparency
- Ethical concerns and accountability
- Job displacement and workforce transformation
- Security and privacy risks
Current Trends and Advancements
The AI landscape is rapidly evolving, with current trends and advancements including:
- Edge AI and distributed intelligence
- Explainable AI (XAI) and transparent AI
- Adversarial AI and robustness
- Transfer learning and meta-learning.
- Autonomous systems and human-AI collaboration
As AI continues to advance and become more pervasive, it’s essential to address the challenges and limitations while harnessing its potential to transform industries and societies for the better.
The Future of AI: Trends and Projections
As AI continues to evolve and improve, it’s essential to look ahead and anticipate the trends and projections that will shape its future. Here are some potential developments and advancements that could transform the AI landscape:
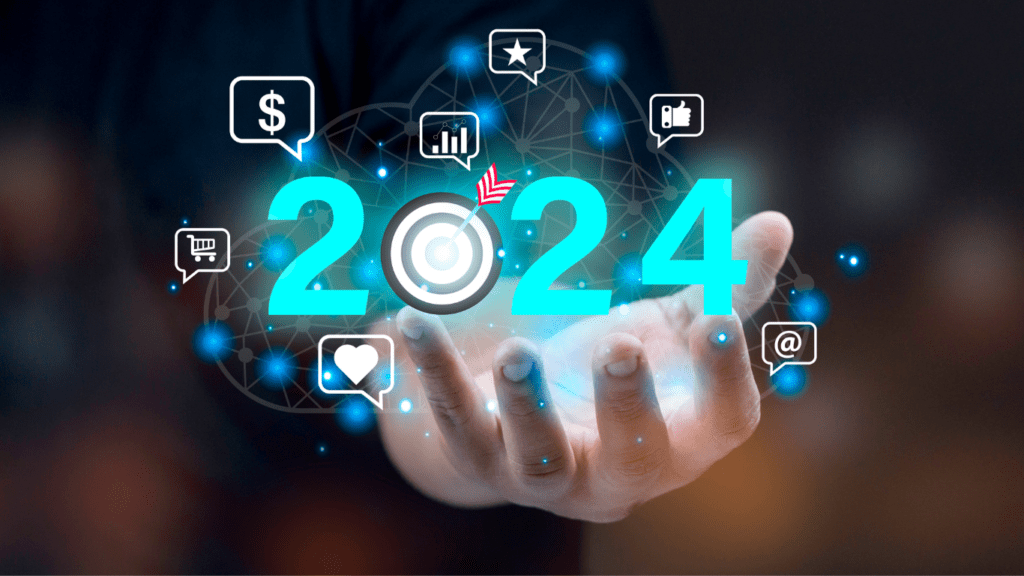
Edge AI and Distributed Intelligence
Edge AI refers to the deployment of AI algorithms and models at the edge of the network, closer to the source of the data. This approach enables faster processing, reduced latency, and improved real-time decision-making. Distributed intelligence, on the other hand, involves the distribution of AI capabilities across multiple devices and nodes, enabling more efficient and scalable processing.
Explainable AI (XAI) and Transparent AI
Explainable AI (XAI) aims to make AI decision-making more transparent and interpretable, enabling users to understand the reasoning and logic behind AI-driven outputs. Transparent AI takes this a step further, providing insights into the AI system’s decision-making processes and enabling users to trust AI outputs more confidently.
Adversarial AI and Robustness
Adversarial AI involves the development of AI systems that can withstand and counter adversarial attacks, ensuring the security and integrity of AI-driven systems. Robustness refers to the ability of AI systems to perform consistently and accurately, even in the face of uncertainty, noise, or errors.
Transfer Learning and Meta-Learning
Transfer learning enables AI models to leverage pre-trained knowledge and adapt to new tasks and domains, reducing training time and improving performance. Meta-learning takes this a step further, enabling AI models to learn how to learn and adapt to new tasks and domains more efficiently.
Autonomous Systems and Human-AI Collaboration
Autonomous systems, such as self-driving cars and drones, will continue to advance, enabling more efficient and effective decision-making. Human-AI collaboration will become more prevalent, enabling humans and AI systems to work together more effectively and efficiently.
AI for Social Good
AI will increasingly be applied to address societal challenges, such as climate change, healthcare, education, and accessibility. AI for social good initiatives will become more prominent, enabling AI to drive positive change and improve lives.
These trends and projections highlight the potential of AI to transform industries and societies. As AI continues to evolve, it’s essential to prioritize responsible AI development, ensuring that AI is developed and deployed ethically, transparently, and accountably.
Conclusion
In conclusion, the dawn of the Age of AI has brought about a transformative shift in the way we live, work, and interact with each other. AI’s potential to revolutionize industries, improve lives, and solve complex problems is vast and exciting. However, it’s crucial to acknowledge the challenges and limitations that come with this technology.
As we move forward, it’s essential to prioritize responsible AI development, ensuring that AI is developed and deployed ethically, transparently, and accountably. This requires a collaborative effort from governments, industries, academia, and individuals to address concerns around data privacy, bias, job displacement, and security.
Moreover, we must recognize the importance of human-AI collaboration, harnessing the strengths of both humans and machines to create a harmonious and productive partnership. By doing so, we can unlock the full potential of AI and create a brighter future for all.
The future of AI is uncertain, but one thing is clear – it has the potential to transform our world in ways we never thought possible. Let’s embrace this technology with caution, curiosity, and creativity, and work together to create a future where AI enhances our lives without compromising our values and humanity.
Final Thoughts
As we conclude this chapter, we’re reminded that the Age of AI is not just about machines; it’s about us – our relationships, our work, our healthcare, our education, and our very existence. It’s a call to action, urging us to be aware, adaptable, and proactive in shaping the future of AI.
Let’s embrace this transformative journey and work together to create a future where AI augments our capabilities, enhances our lives, and inspires us to reach new heights. The Age of AI has dawned, and it’s up to us to seize its potential and create a brighter future for all.
Further Reading
- #Artificial Intelligence Revolution
- #AI Technology Trends
- #Machine Learning Applications
- #Deep Learning Algorithms
- #Natural Language Processing Techniques
- #Computer Vision Systems
- #Autonomous Systems Development
- #Data Science and Analytics
- #Big Data Processing
- #Internet of Things (IoT) Solutions
- #Cloud Computing Services
- #Edge AI Solutions
- #Explainable AI (XAI) Techniques
- #Transparent AI Systems
- #Adversarial AI Attacks
- #Robust AI Development
- #Transfer Learning Methods
- #Meta-Learning Algorithms
- #Human-AI Collaboration Tools
- #Autonomous Vehicle Technology
- #AI in Healthcare Industry
- #AI in Education Sector
- #AI Employment Opportunities
- #Ethical AI Development
- #AI Bias and Privacy Concerns
- #AI Security Measures
Explore Micro2media.com
- Unveiling the Power of AI in Mathematics: Revolutionizing Problem-solving and Discovery
- Unlocking the Future of Shopping: Exploring Consumer Intentions at AI-Powered Automated Retail Stores (AIPARS)
- A Root Revolution: AI Empowers Plants to Fight Climate Change
- Revolutionizing Oncology: How AI is Transforming Cancer Treatment
- A Landmark Achievement: Engineering Artificial Cells that Mimic Life
- Unraveling the Wonders of Artificial Neural Networks: A Deep Dive into Machine Learning’s Brainpower
- Revolutionizing Literacy: How Artificial Intelligence is Transforming Computer-Based Reading and Writing Instruction
- Decoding the Future: Navigating the Crossroads of Cryptocurrencies and Artificial Intelligence
- Judgment Day: Navigating the Pros and Cons of AI in Courtrooms
- Rolling the Dice: Navigating Probability Judgement in Artificial Intelligence
- Revolutionizing Tomorrow: The Rise of ChatGPT and the Future of AI-Powered Conversations
- AI-fueled Freelancing: Revolutionizing Work in the Gig Economy
- Empowering Minds: How Students are Harnessing AI for Innovation – Exploring the Pros and Cons
- Unlocking Precision: The AI Revolution in Software Quality Assurance